š§ Integrating AI into iOS Apps: Using CoreML + Swift
20 April 2025
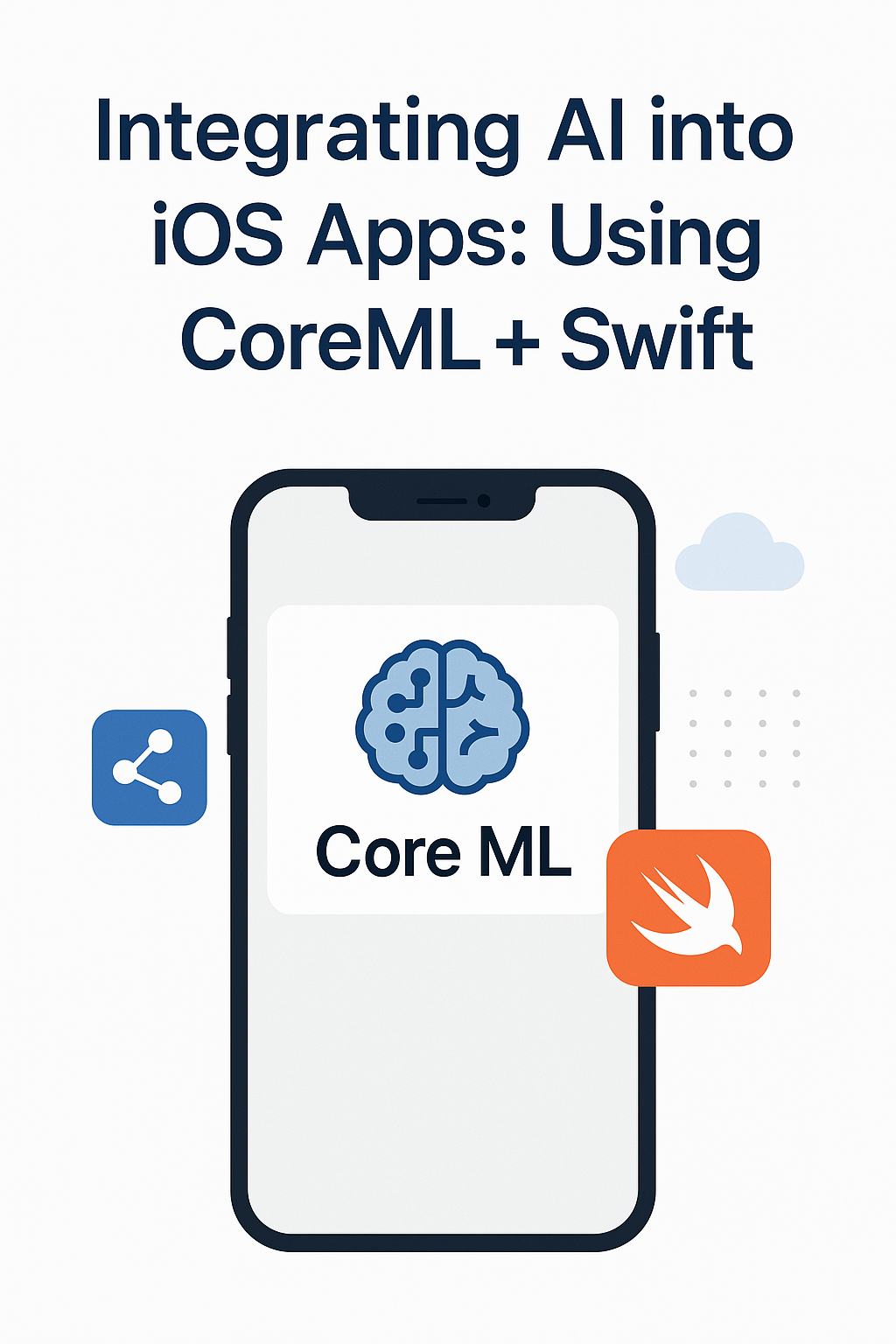
Artificial Intelligence is rapidly transforming mobile app developmentāand iOS is no exception. Apple has made integrating machine learning models seamless with CoreML, allowing developers to bring intelligent features right into their apps using Swift.
In this post, weāll explore:
- What CoreML is
- How to use it in your iOS app
- A quick walkthrough using a sample ML model
š What is CoreML?
CoreML is Appleās machine learning framework, optimized for on-device performance and privacy. You can use it to:
- Classify images
- Predict user behavior
- Recognize speech or text
- Perform natural language processing
- And more!
It supports popular model types like .mlmodel, and can convert models from frameworks like TensorFlow, PyTorch, or scikit-learn.
š§° How to Use CoreML in Swift
Letās break it down into 3 main steps:
1. Get an ML Model
You can:
- Build your own model and convert it using coremltools
- Download a pre-trained model from Appleās Model Gallery
- Use Create ML to build your own custom model
Example: Letās use MobileNetV2.mlmodel to classify images.
2. Add the Model to Your Project
- Drag the .mlmodel file into your Xcode project.
- Xcode will auto-generate a Swift class for the model.
Example:
Swift
let model = try! MobileNetV2(configuration: MLModelConfiguration())
3. Use the Model in Code
Hereās a sample method to classify an image:
Swift
func classifyImage(_ image: UIImage) {
guard let pixelBuffer = image.toCVPixelBuffer() else { return }
do {
let prediction = try model.prediction(image: pixelBuffer)
print("Prediction: \(prediction.classLabel)")
} catch {
print("Failed to predict: \(error)")
}
}
š§ Note: Youāll need an extension to convert UIImage to CVPixelBufferāavailable on GitHub or Apple's docs.
š§Ŗ Testing & Debugging
Always test your modelās performance:
- On various devices (CoreML runs faster on newer chips)
- With edge cases (e.g., blurry or unusual images)
- Using real-world data instead of only training sets
š Benefits of On-Device AI
- Privacy: Data never leaves the device
- Speed: No network latency
- Offline Access: Works without an internet connection
š¦ Final Thoughts
Integrating AI in iOS apps using CoreML + Swift is not just possibleāitās powerful and beginner-friendly. With a few lines of code, you can turn your app into a smart, adaptive experience for users.
Whether you're building a photo classifier, language translator, or custom recommender systemāCoreML is your gateway to adding real-time intelligence to your iOS app.